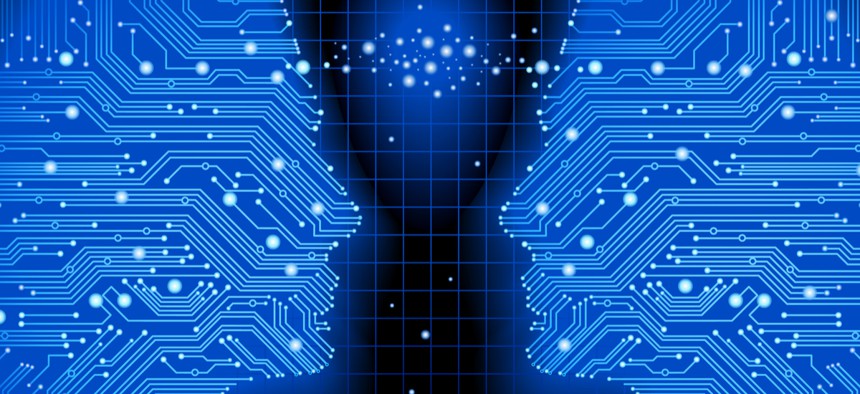
Social Science? Data Science? Evidence-Based Government Needs Both
Results-focused leaders need to get savvy about both if they want to catalyze a culture of continuous improvement.
Let’s say you’re a public-sector chief executive, or an agency or program leader who cares about building a culture of data-driven decision-making. In working to strengthen organizational capacity, should you prioritize:
- Traditional social science, such as rigorous program evaluation, that typically uses custom-made datasets (created for a particular study) to credibly answer research questions?
- Or data science, such as data mining, predictive modeling and artificial intelligence that can use ready-made datasets (data already collected for a different purpose) and repurposes it to inform data-driven decision making?
I’d suggest using both, simultaneously. In fact, results-focused government today requires digital-age social science, which is a hybrid between traditional social science and more cutting-edge data science. It recognizes the strengths and weaknesses of each approach and the synergies between them.
Traditional social science, for example, can be expensive, involving the collection of survey data from sometimes thousands of sample members. Yet customized data is very helpful for answering specific research questions. Data science, on the other hand, uses much cheaper existing data—often called “big data.” But overlooking the limits of repurposing data can easily produce meaningless correlations, not useful insights.
Digital-age social science uses both approaches smartly. It means that social scientists who want to answer research questions as accurately, quickly and efficiently as possible need to pay attention to all of the ready-made data that exists today. And data scientists who want to produce impactful research need to work with government officials to identify the most important problems or questions to be examined.
What does digital-age social science look like in practice? Several examples were provided at a panel this month organized by the Association of Policy Analysis and Management and Mathematica Policy Research.
- Kansas City is using predictive modeling to determine which property-code violations likely need more attention to resolve.
- The National Cancer Institute’s SmokeFree.gov initiative is using artificial intelligence to create a chatbot (a computer program designed to simulate conversation) that provides live counseling, walking the user through what it takes to quit smoking.
- Researchers measuring poverty in Rwanda mixed custom-made data (survey data) with ready-made data (anonymized cell phone data) to get national poverty estimates in one-tenth of the time at one-fiftieth of the cost.
- The federal government’s Office of Evaluation Sciences has been helping agencies embed quick, low-cost randomized experiments—what private sector companies calls A/B testing—into their programs to inform operational improvements.
To help your organization capture the benefits of both social science and data science, I have four suggestions, drawn from insights from the panel:
First, researchers in your agency (or ones with whom you partner or contract) should ask the following question: If I’m primarily using a ready-made or custom-made data set, is there some data set of the other kind that can enrich the data I have? It is a question that underscores how the analytical approaches complement each other.
Second, a simple but useful way to combine social science and data science perspectives is to invite both types of staff into the room—literally—to discuss key challenges or opportunities facing your organization. Their real-time interchange of ideas will lead to shared approaches and also help address potential weaknesses of each perspective. Social scientists, for example, can help data scientists avoid using problematic data or poorly expressed research questions. Data scientists can help traditional social scientists avoid costly primary data collection efforts when existing high-quality data sets might be repurposed for the job.
Third, always start by identifying the key organizational problems or opportunities you want to address before thinking about methods. As Bob Behn of the Harvard Kennedy School has put it, “Always start with purpose.” Creating a learning agenda can be a useful way to do that. It ensures that evidence-building resources, whether using social science or data science, are used as efficiently as possible.
Finally, allow staff with valuable, innovative ideas to use some of their time to develop and pilot those ideas, with access to experts in social science and data science who can help them do that. The Data Science CoLab at the U.S. Department of Health and Human Services is a good example.
The fact is, both social science and data science and here to stay. One uses proven methods from the past that are still valuable today. The other draws on the proliferation of new digital data sources, as well as the increased computer power that can quickly make sense of massive volumes of data, to create new opportunities for research. Results-focused leaders need to get savvy about both if they want to catalyze a culture of continuous learning and improvement.
Andrew Feldman is a director in the public-sector practice at Grant Thornton. He was previously a visiting fellow at the Brookings Institution and a special adviser on the evidence team at the White House Office of Management and Budget.