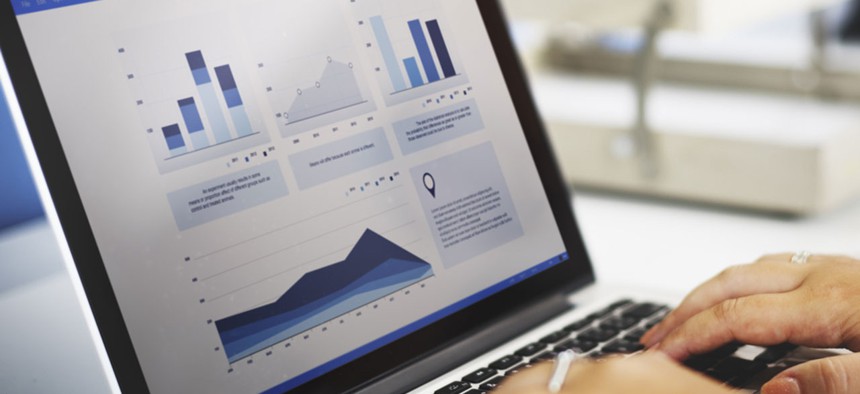
Rawpixel.com/Shutterstock.com
How Statistics are Twisted to Obscure Public Understanding
Statistics are often used to support points that aren’t true, but we tend to attack only the data that conflict with some preexisting notion of our own.
Mark Twain attributed to Benjamin Disraeli the famous remark: ‘There are three kinds of lies: lies, damned lies, and statistics.’ In every industry, from education to healthcare to travel, the generation of quantitative data is considered important to maintain quality through competition. Yet statistics rarely show what they seem.
If you look at recent airline statistics, you’ll think that a far higher number of planes are arriving on schedule or early than ever before. But this appearance of improvement is deceptive. Airlines have become experts at appearance management: by listing flight times as 20-30 per cent longer than what the actual flight takes, flights that operate on a normal to slightly delayed schedule are still counted as arriving ‘early’ or ‘on time’. A study funded by the Federal Aviation Administration refers to the airline tactic as schedule buffering.
It is open to question, however, whether flights operating on a buffered schedule arrive ‘on time’ in the sense that ordinary people use the term. If a flight is scheduled for 2.5 hours and takes, on average, only 1.5 hours to reach its destination, then is any flight that arrives at its scheduled time really on time? Or have the airlines merely redefined the term ‘on time’ to generate more favourable statistics?
This example of airlines twisting meaning – and, consequently, public perception – might be irritating, but it is by no means the only industry where semantic manipulation of statistics is found. University rankings are especially prominent: numerous publications rank universities on a variety of metrics, relying on factors such as a university’s acceptance rate, average student test scores and job placement, to name a few.
But in recent years the competition among universities has become so intense that several have admitted to dishonestly manipulating the stats. In August 2012, Emory University admitted, after an internal investigation, that the administration had misreported incoming students’ test scores for a decade. And Emory was not the only culprit: in 2013, Forbes magazine removed three other major colleges from its rankings for similar infractions. The quantitative data weighed by rankings publications determine how we, ordinary people, understand phrases such as ‘the best universities’. But how can a system that rewards semantical manipulation be said to explain where students receive the best education?
Similar problems plague the healthcare system in the United States. An important concept for ranking hospitals is ‘survivability’, which the US News & World Report defines as ‘30 days after hospitalisation, adjusted for severity’. Avery Comarow, health rankings editor at US News, said in an email that ‘30-day mortality is the most common benchmarking period used by researchers, insurers and hospitals themselves for evaluating in-hospital mortality, because it recognises that a hospital is responsible for patients not just during their hospital stay but for a reasonable period after they are discharged’.
But what if a group of patients lives only for 32 days after hospitalisation? Ordinary people do not think of ‘survival’ as 30 days after any event; why should we trust a ranking system that uses such a fundamental and important notion in an unrecognisable way? Furthermore, does this definition favour hospitals that choose not to admit patients believed unlikely to live until the 30-day mark? What implicit pressures are placed on hospitals when society relies on the statistical analysis of a ranking publication as a guide to quality?
Writing in the journal Statistical Science, the sociologist Joel Best argues that we ought to avoid calling statistics ‘lies’, and instead educate ourselves so that we can question how and why statistical data are generated. Statistics are often used to support points that aren’t true, but we tend to attack only the data that conflict with some preexisting notion of our own. The numbers themselves – unless purposefully falsified – cannot lie, but they can be used to misrepresent the public statements and ranking systems we take seriously. Statistical data do not allow for lies so much as semantic manipulation: numbers drive the misuse of words. When you are told a fact, you must question how the terms within the fact are defined, and how the data have been generated. When you read a statistic, of any kind, be sure to ask how – and more importantly, why – the statistic was generated, whom it benefits, and whether it can be trusted.
Jonathan R Goodman is a postgraduate researcher at the department of biology at Queens College, CUNY, and an editor at the Cancer Therapy Advisor. He is interested in the relationship between biology and ordinary language.
This article was originally published at Aeon and has been republished under Creative Commons.